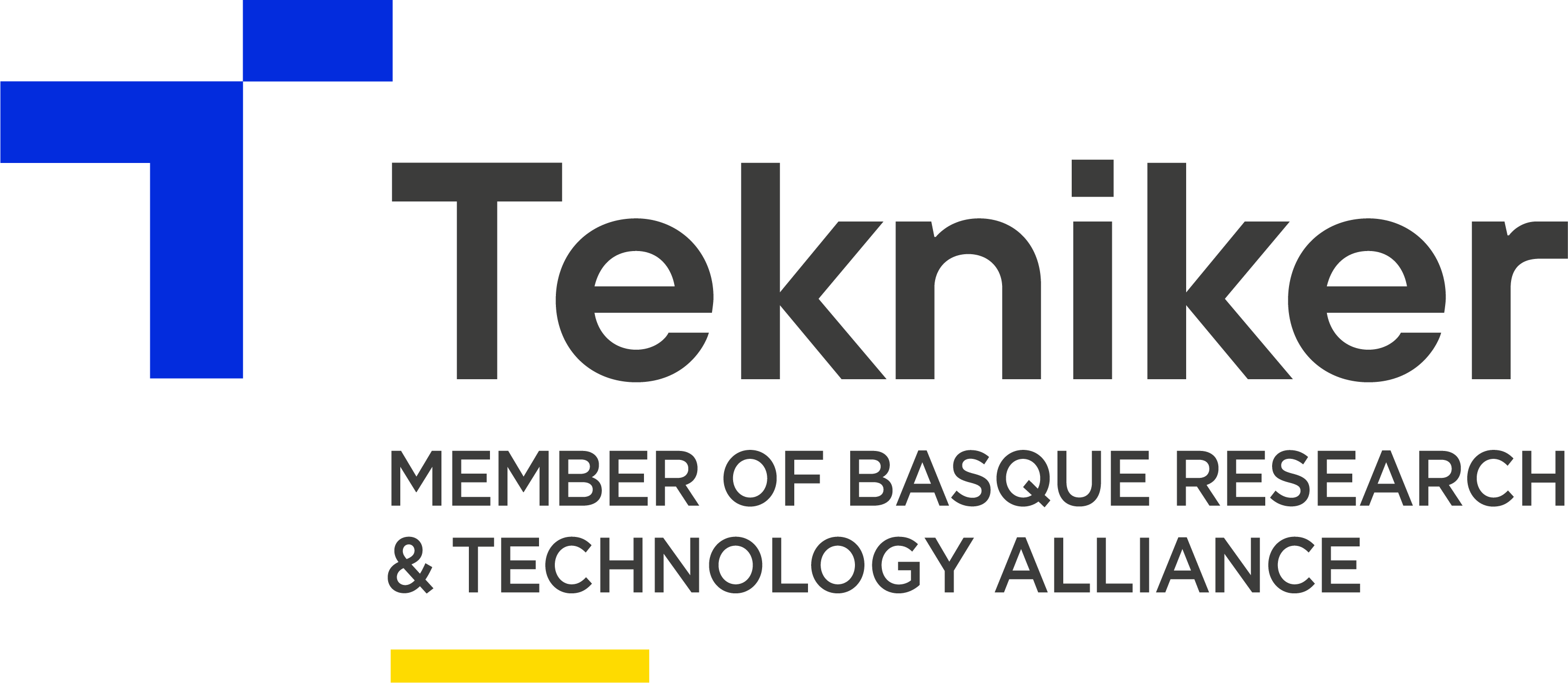
Tekniker – Machine learning and deep learning
Tekniker
Sector: Construction
Business Case
In construction environments where mobile machines operate, there is a high risk of accidents due to the interaction between humans and heavy machinery. Challenges include variability in environmental conditions (such as light and weather), the complexity and dynamism of the environment, and the need to accurately detect people and objects to avoid collisions or accidents. The efficacy of conventional solutions is limited by these conditions giving rise to a large number of false positives, raising the need for more advanced and reliable technology.
Objectives
The project addresses the difficulty of the scarcity of public people-centric datasets in construction environments to create robust artificial intelligence models, which is crucial for accurate, real-time tracking of workers. In addition, it was a challenge to develop a solution that reduces false positives in various work situations, such as when workers are near heavy machinery. Overcoming these obstacles is essential to achieve the main objective of the project: to implement an advanced monitoring system that not only alerts in a personalised way but also acts on the machinery, stopping it automatically in dangerous situations to ensure maximum safety in the workplace.
Use case
The project approach combines RGB-D camera imagery and artificial intelligence to strengthen construction safety, with AI models trained to detect and track people on construction sites accurately. Worker intentions are assessed by analysing their trajectory and point cloud information is used to understand the environment, synchronising this data with the operation of the machinery. This not only allows proactive anticipation of potential incidents, issuing customized alerts and reducing false positives, but also enables a direct and automatic response on the machinery, stopping it if necessary to prevent dangerous situations.
Infrastructure
Solution: Edge Training: On Premise and Cloud
Technology
Image recognition/processing Automatic or Deep Learning
Data
Own dataset generated and labelled in different PAVASAL works.
Resources
At the organisational level, the development required 1 project manager, 4 computer vision and AI specialists and 1 hardware technician. Technical development and validation by Tekniker and PAVASAL.
Difficulties and learning
The project encountered the obstacle of the scarcity of public datasets of people in construction environments, essential for generating robust and accurate artificial intelligence models. This challenge was compounded by the need to develop a solution capable of minimising false positives, especially in scenarios where workers are operating near heavy machinery, where accurately distinguishing between routine movements and hazardous behaviours is critical to site safety.
KPIs (business impact and metrics of the model)
mAP, Accuracy, Recall and proprietary metrics
Funding
This project obtained funding through the programme “Research and Development Projects on Artificial Intelligence and other digital technologies and their integration into value chains” of the public business entity Red.es, the European Regional Development Fund (ERDF) “A way of making Europe”.
Collaborators, Partners
PAVASAL