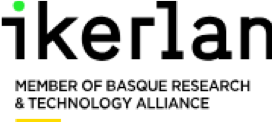
Ikerlan – IA4BAT - Optimisation and Artificial Intelligence for Real-Time Second Life Battery Operation
Ikerlan
Sector: Energy
Business Case
Energy storage plays a vital role in tackling the energy transition challenge. It enhances the management of renewable energy production and ensures the stability of the electricity system as intermittent renewable technologies become more prevalent in the coming decades. In this scenario, renewable plants will have to optimise their participation in electricity markets, provide additional services to maintain security and quality of supply, and improve their real-time operation. All this can be carried out thanks to battery installation and optimal operation.
Objectives
The objective of this project is to serve as a demonstration and validation of energy storage in representative environments and real operating conditions, such as the renewable generation plants managed by Capital Energy. Electrical storage, understood as an energy vector, will enable the manageability and operation of renewable generation and will provide them with technical improvements and new features that are not currently in place. For the development of the battery module, Capital Energy worked on an AI platform using machine learning techniques, which was integrated and validated in the Cloud GCP platform.
Use case
The proposed solution involves the integration of two second life storage systems in a renewable plant managed by Capital Energy. To efficiently handle these two storage systems and enhance their involvement in the electricity markets of the renewable plant, three dedicated artificial intelligence modules were created. These modules address battery degradation, predict electricity market prices, and optimise market operations, respectively.
Infrastructure
Cloud On Premise
Technology
Machine learning and deep learning
Data
Private of battery degradation datasets. Public datasets (historical data from electricity markets) together with private datasets (estimates of future electricity markets).
Resources
Multidisciplinary team of researchers specialised in AI model development and with extensive knowledge of storage systems and electricity markets. Equipment with high-performance computing capabilities for training AI models and optimising the participation of renewable plants with storage in electricity markets.
Difficulties and learning
Challenges arose in obtaining degradation data from storage systems and acquiring historical electricity market data, but especially for future estimates. Finding highly qualified personnel both in the development of AI models and with extensive knowledge of storage systems and electricity markets.
KPIs (business impact and metrics of the model)
Capital Energy expects to avoid the emission of more than 575 tons of CO2 into the atmosphere from two main sources: the integration of renewables into the electricity system and the use of second-life batteries. In addition, by optimising participation in electricity markets, the profitability of new renewable generation plants with storage systems is expected to improve.
Funding
The project was funded thanks to the support of RED.ES and the European Regional Development Fund (ERDF), with file number 2020/0720/00099631.
Collaborators, Partners
Capital Energy, Ikerlan S. Coop, BeePlanet Factory, Google Cloud Platform, CIC Consulting