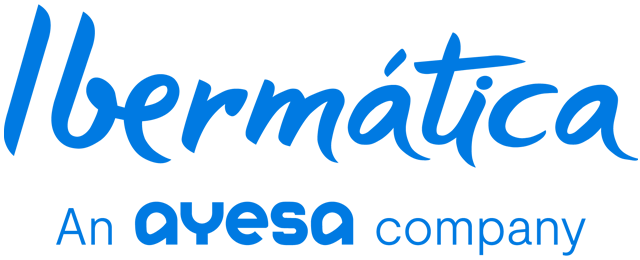
Ayesa Ibermática fundazioa – Optimisation of the reassignment of sales positions to workers with Quantum Computing.
Ayesa Ibermática fundazioa
Sector: Mobility
Business Case
Management of a large number of reassignments of sales staff to positions, due to numerous, often unforeseen circumstances. Classical optimisation solutions to complex combinatorial problems are computationally expensive and offer no guarantee of obtaining the optimal solution, since they do not evaluate all possible scenarios.
Objectives
Obtain a solution with the certainty that the results obtained are the most accurate, specific and efficient. Improve both the number of assignments to positions and the gain of assigned positions in terms of their importance. Maintain the conditions of restrictions in terms of the particular context of each salesperson, distance to the position, availability, etc.
Use case
In algorithmic terms, the problem was defined as a “QUBO” problem, in which both the function to be minimised and the constraints were encoded in the respective Hamiltonians. An analytical model was also developed to include in the model a preference for assigning salespeople to positions, based on previous assignment histories. The trade-off between historical assignment support (times a salesperson has been assigned to a position historically), and the gain in assigning salespeople to “novel” positions, is performed by a trade-off coefficient analysis. This project also implemented a quantum machine learning solution, or QML, capable of modelling the manager’s subjective behaviour based on the historical data obtained, and automatically replicating the subjective assignment of salespeople based on the context (day, month, billboard, etc.).
Infrastructure
Cloud
Technology
– Quantum – Automatic or deep learning
Data
Private datasets
Resources
Quantum Computational Scientist – Quantum Inspire Expert , Quantum Optimization Expert, Quantum ML Ingenieros de datos, Diseñadores de Aplicaciones.
Difficulties and learning
Learning about the QOPS methodology developed by Ayesa and Ibermática Fundazioa to support quantum computing projects in real environments. For this purpose, different solutions were analysed, but none of the current quantum cloud platforms achieved the desired results. A proprietary quantum solver has been developed to solve combinational problems using Polynomial Unconstrained Binary Optimisation (PUBO) methods.
KPIs (business impact and metrics of the model)
The assignment process for absenteeism cases is done in 15 minutes, when previously it required at least 2 hours to assign. The automatically proposed assignments improve both the number of assignments, doubling on average, and tripling the assignment scoring based on the weight of the positions filled. The system allows “predictive” assignments to be generated, in the future (20 days each day). The work of the technical staff is only subject to the approval of the recommendations by the quantum system, assuring them that, thanks to the quantum “brute force” analysis technology, the proposed scenario is the best one, so the effectiveness, workload and safety for the technical staff in their daily work, has been a very important improvement.
Funding
No
Collaborators, Partners
ONCE Innovation Lab